Deep Learning: Tackling the challenges of bioimage analysis
Deep learning has shown promising results in a wide range of imaging problems in recent years (LeCun et al., 2015), and has the potential to help researchers by automating the analysis of various kinds of biological images (Ronneberger et al., 2015). However, many deep-learning methods require a large amount of 'training data' in order to produce useful results, and this is often not available for bioimage analysis. There is, therefore, a need for deep-learning methods that can make the most from a limited amount of training data. Now, in eLife, Robert Blum (University Hospital Würzburg), Christoph Flath (University of Würzburg) and colleagues – including Dennis Segebarth and Matthias Griebel as joint first authors – provide guidance on how to do this in bioimage analysis (Segebarth et al., 2020).
A common approach to applying deep learning to image analysis involves 'convolutional neural networks': these networks take an input image (such as a microscopy image) and perform many mathematical operations on it to produce an output image (such as a corresponding image with interesting features annotated). A convolutional neural network is characterized by a set of 'learnable parameters', which have to be set to the correct values for the network to perform a given task. The act of finding the correct values for these parameters is called 'training', and several different training techniques are used in practice.
In supervised learning, training is performed using a set of input images and target output images, and the learnable parameters are iteratively adjusted until the output images produced by the network match the target images. It is important to note that supervised learning involves a large amount of randomness, and that training multiple networks using the same data will result in different networks that produce (slightly) different output images.
In bioimage analysis, a common task is to annotate certain structures in images produced by techniques such as microscopy, cryo-EM or X-ray tomography (Meijering et al., 2016). However, the complicated nature of biological images means that this annotation often has to be done by a human expert, which is time-consuming, labour-intensive and subjective (Figure 1A). Supervised deep learning could provide a way to automate the annotation process, reducing the burden on human experts and enabling analysis of a significantly larger set of images. However, annotating the input images needed to train the network also requires a significant amount of time and effort from a human expert.
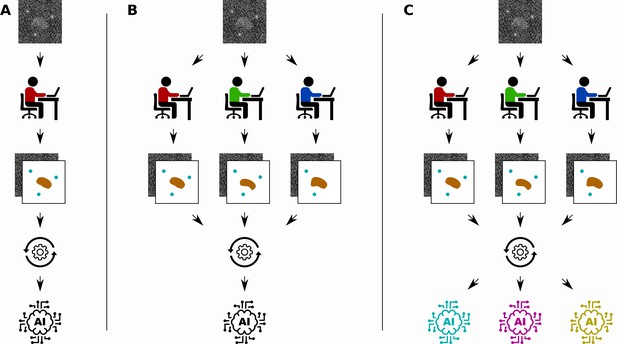
Different ways to train a convolutional neural network.
Segebarth et al. compare three techniques for training convolutional neural networks to analyze bioimages. (A) In the standard approach a single human expert annotates images for training a single network. (B) In a second approach multiple human experts annotate the same images, and consensus images are used for training: this improves the objectivity of the trained network. (C) In a third approach, a technique called model ensembling is added to the second approach, meaning that multiple networks are trained with the same consensus images: this improves the reliability of the results.
Several properties of a trained network are important for real-world applications. The first is its objectivity, referring to a lack of influences from the subjective nature of human annotations. The second is its reliability, meaning that the trained network should consistently annotate similar features in the same way. The third is its validity, referring to the truthfulness of the network output (that is, did we annotate what we intended to?). In practice, it is often the case that objectivity, reliability and validity are difficult to achieve when the amount of training data is limited.
Various approaches to improve the objectivity, reliability and validity of convolutional neural networks have been proposed. Some involve adapting the structure of the network themselves by, for example, reducing the number of learnable parameters (Pelt and Sethian, 2018), and some involve adapting the training method by, for example, randomly ignoring parts of the network during training (Srivastava et al., 2014). A different approach is to focus on the training data used in supervised learning. Given a network structure and a training method, how can the training data set be optimized to improve objectivity, reliability and validity? In other words, given the time-consuming, labour-intensive and subjective nature of manual annotation, how can a limited period of time from human experts be best utilized to produce a training data set? These questions are currently the subject of active research.
Segebarth et al. investigate two techniques for improving the objectivity, reliability and validity of trained convolutional neural networks in bioimage analysis. First, they investigate the use of multiple human experts to annotate the same set of training images (Figure 1B). The different annotations of each input image are then combined to create a consensus target output image. Since each human expert has their own intended and unintended biases, networks that are trained with data from a single human expert might include the biases of the expert. Using consensus images from multiple experts during training can improve the objectivity of the resulting networks by removing these biases from the training data.
The second technique is to train multiple convolutional neural networks using the same training data set, and then combine the results when the networks are used to analyse new images (Figure 1C). This technique, called model ensembling, has already proven successful in a wide range of applications (Krizhevsky et al., 2017). Model ensembling is based on the randomness involved in training described above: because of this randomness, each trained network will be implicitly biased in their results. By combining the output of multiple networks, these biases are effectively removed, resulting in more reliable results.
A key contribution of Segebarth et al. was to perform extensive experiments on real images and show that the use of consensus images and model ensembles does indeed improve objectivity, reliability and validity. This provides a recipe for optimizing the generation of training data and for making efficient use of the available data, although this recipe still requires a significant amount of human expert time since each image has to be annotated by multiple experts. The results could also help researchers trying to understand how biases affect trained networks, which could lead to improved network structures and training approaches (Müller et al., 2019). And although many questions and challenges remain, the work of Segebarth et al. represents an important step forward in the effort to make the use of deep learning in bioimage analysis feasible.
References
-
ImageNet classification with deep convolutional neural networksCommunications of the ACM 60:84–90.https://doi.org/10.1145/3065386
-
Imagining the future of bioimage analysisNature Biotechnology 34:1250–1255.https://doi.org/10.1038/nbt.3722
-
ConferenceWhen does label smoothing help?Advances in Neural Information Processing Systems. pp. 4694–4703.
-
BookU-net: Convolutional networks for biomedical image segmentationIn: Navab N, Hornegger J, wells W, Frango A, editors. International Conference on Medical Image Computing and Computer-Assisted Intervention. Springer. pp. 234–241.https://doi.org/10.1007/978-3-319-24574-4_28
-
Dropout: a simple way to prevent neural networks from overfittingJournal of Machine Learning Research 15:1929–1958.
Article and author information
Author details
Publication history
- Version of Record published: December 2, 2020 (version 1)
Copyright
© 2020, Pelt
This article is distributed under the terms of the Creative Commons Attribution License, which permits unrestricted use and redistribution provided that the original author and source are credited.
Metrics
-
- 1,139
- views
-
- 110
- downloads
-
- 5
- citations
Views, downloads and citations are aggregated across all versions of this paper published by eLife.
Download links
Downloads (link to download the article as PDF)
Open citations (links to open the citations from this article in various online reference manager services)
Cite this article (links to download the citations from this article in formats compatible with various reference manager tools)
Further reading
-
- Computational and Systems Biology
Transcriptomic profiling became a standard approach to quantify a cell state, which led to accumulation of huge amount of public gene expression datasets. However, both reuse of these datasets or analysis of newly generated ones requires significant technical expertise. Here we present Phantasus - a user-friendly web-application for interactive gene expression analysis which provides a streamlined access to more than 96000 public gene expression datasets, as well as allows analysis of user-uploaded datasets. Phantasus integrates an intuitive and highly interactive JavaScript-based heatmap interface with an ability to run sophisticated R-based analysis methods. Overall Phantasus allows users to go all the way from loading, normalizing and filtering data to doing differential gene expression and downstream analysis. Phantasus can be accessed on-line at https://alserglab.wustl.edu/phantasus or can be installed locally from Bioconductor (https://bioconductor.org/packages/phantasus). Phantasus source code is available at https://github.com/ctlab/phantasus under MIT license.
-
- Computational and Systems Biology
- Evolutionary Biology
A comprehensive census of McrBC systems, among the most common forms of prokaryotic Type IV restriction systems, followed by phylogenetic analysis, reveals their enormous abundance in diverse prokaryotes and a plethora of genomic associations. We focus on a previously uncharacterized branch, which we denote coiled-coil nuclease tandems (CoCoNuTs) for their salient features: the presence of extensive coiled-coil structures and tandem nucleases. The CoCoNuTs alone show extraordinary variety, with three distinct types and multiple subtypes. All CoCoNuTs contain domains predicted to interact with translation system components, such as OB-folds resembling the SmpB protein that binds bacterial transfer-messenger RNA (tmRNA), YTH-like domains that might recognize methylated tmRNA, tRNA, or rRNA, and RNA-binding Hsp70 chaperone homologs, along with RNases, such as HEPN domains, all suggesting that the CoCoNuTs target RNA. Many CoCoNuTs might additionally target DNA, via McrC nuclease homologs. Additional restriction systems, such as Type I RM, BREX, and Druantia Type III, are frequently encoded in the same predicted superoperons. In many of these superoperons, CoCoNuTs are likely regulated by cyclic nucleotides, possibly, RNA fragments with cyclic termini, that bind associated CARF (CRISPR-Associated Rossmann Fold) domains. We hypothesize that the CoCoNuTs, together with the ancillary restriction factors, employ an echeloned defense strategy analogous to that of Type III CRISPR-Cas systems, in which an immune response eliminating virus DNA and/or RNA is launched first, but then, if it fails, an abortive infection response leading to PCD/dormancy via host RNA cleavage takes over.