Experience transforms crossmodal object representations in the anterior temporal lobes
Figures
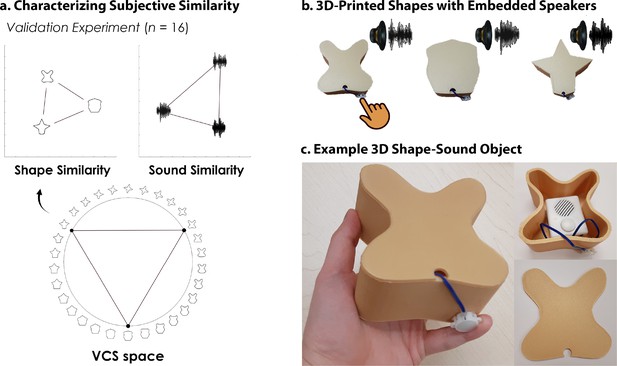
3D-printed objects.
An independent validation experiment ensured that the similarity of the selected shapes and sounds were well-matched. (a) Three shapes were sampled from the Validated Circular Shape (VCS) Space (shown as black points on VCS space), (Li et al., 2020) a stimulus space whereby angular distance corresponds to subjective shape similarity. Three sounds were sampled from a set of five experimenter-created sounds. This independent validation experiment ensured that we could characterize the change in similarity structure following crossmodal learning, because we knew the baseline similarity structure that is, two triangular representational geometries visualized using multidimensional scaling (Shepard, 1980; also see Figure 2—figure supplement 1). Furthermore, this procedure ensured that the subjective similarity of the three features was equated within each modality. (b) The shapes were then 3D-printed with a hollow space and embedded with a button-activated speaker. (c) Participants could physically explore and palpate the 3D shape-sound objects. Critically, we manipulated whether the button-activated speaker was operational across learning days (see Methods/Figure 2).
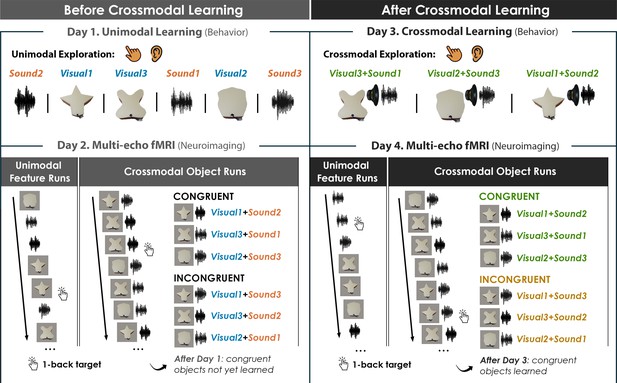
Four-day crossmodal object learning task.
On Day 1 (behavior), participants heard sounds through a headset and explored 3D-printed shapes while the button-activated speakers were not operational. During a separate task (Figure 2—figure supplement 1), participants rated the similarity of the visual shapes and sound features. On Day 2 (neuroimaging), participants completed (i) 10 Unimodal Feature runs in which they performed a 1-back task involving the shape and sound features experienced separately and (ii) 5 Crossmodal Object runs in which they performed a 1-back task for the shapes and sounds experienced simultaneously. As participants at this point have not yet learned the congruent shape-sound pairings, the Day 2 neuroimaging session serves as a within-subject neural baseline for how the unimodal features were represented before crossmodal learning. On Day 3 (behavior), participants again explored the shape and sound features. Participants now learned to make crossmodal associations between the specific visual and sound features that composed the shape-sound object by pressing the button to play an embedded speaker, thus forming congruent object representations (i.e. crossmodal learning). Shape-sound associations were counterbalanced across participants, and we again collected similarity ratings between the shapes and sounds on a separate task. On Day 4 (neuroimaging), participants completed the same task as on Day 2. In summary, across 4 days, we characterized the neural and behavioral changes that occurred before and after shapes and sounds were paired together to form crossmodal object representations. As the baseline similarity structure of the shape and sound features were a priori defined (see Figure 1) and measured on the first day of learning (see Figure 2—figure supplement 1), changes to the within-subject similarity structure provide insight into whether the crossmodal object representations (acquired after crossmodal learning) differed from component unimodal representations (acquired before crossmodal learning).

Pairwise similarity task and results.
In the initial stimulus validation experiment, participants provided pairwise ratings for five sounds and three shapes. The shapes were equated in their subjective similarity that had been selected from a well-characterized perceptually uniform stimulus space (Li et al., 2020) and the pairwise ratings followed the same procedure as described in Li et al., 2020. Based on this initial experiment, we then selected the three sounds from the that were most closely equated in their subjective similarity. (a) 3D-printed shapes were displayed as images, whereas sounds were displayed in a box that could be played when clicked by the participant. Ratings were averaged to produce a similarity matrix for each participant, and then averaged to produce a group-level similarity matrix. Shown as triangular representational geometries recovered from multidimensional scaling in the above, shapes (blue) and sounds (orange) were approximately equated in their subjective similarity. These features were then used in the 4-day crossmodal learning task. (b) Behavioral results from the 4-day crossmodal learning task paired with multi-echo fMRI described in the main text. Before crossmodal learning, there was no difference in similarity between shape and sound features associated with congruent objects compared to incongruent objects – indicating that similarity was controlled at the unimodal feature-level. After crossmodal learning, we observed a robust shift in the magnitude of similarity. The shape and sound features associated with congruent objects were now significantly more similar than the same shape and sound features associated with incongruent objects (p<0.001), evidence that crossmodal learning changed how participants experienced the unimodal features (observed in 17/18 participants). (c) We replicated this learning-related shift in pattern similarity with a larger sample size (n=44; observed in 38/44 participants). *** denotes p<0.001. Horizontal lines denote the comparison of congruent vs. incongruent conditions.
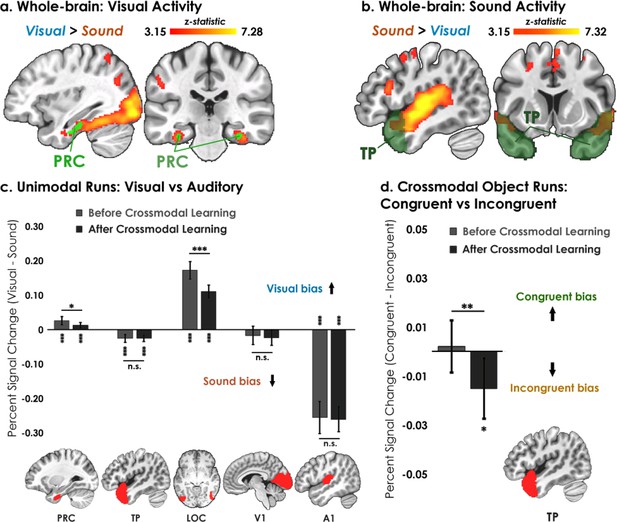
Univariate results.
(a–b) Univariate analyses superimposed on MNI-152 standard space. All contrasts were thresholded at voxel-wise p=0.001 and cluster-corrected at p=0.05 (random-effects, FSL FLAME; 6 mm spatial smoothing). Collapsing across learning days, robust modality-specific activity was observed across the neocortex. (c–d) Five ROIs were a priori selected based on existing theory:Patterson et al., 2007; Cowell et al., 2019 temporal pole – TP, perirhinal cortex – PRC, lateral occipital complex – LOC, primary visual cortex – V1, and primary auditory cortex – A1. (c) Consistent with the whole-brain results, LOC was biased toward visual features whereas A1 and TP were biased toward sound features. Activation in PRC and LOC showed learning-related shifts, with the magnitude of visual bias decreasing after crossmodal learning. (d) TP was the only brain region to show an experience-dependent change in univariate activity to the learned shape-sound associations during crossmodal object runs. * p<0.05, ** p<0.01, *** p<0.001. Asterisks above or below bars indicate a significant difference from zero. Horizontal lines within brain regions reflect an interaction between modality or congruency with learning day (e.g. reduction in visual bias after crossmodal learning in PRC). Error bars reflect the 95% confidence interval (n = 17).
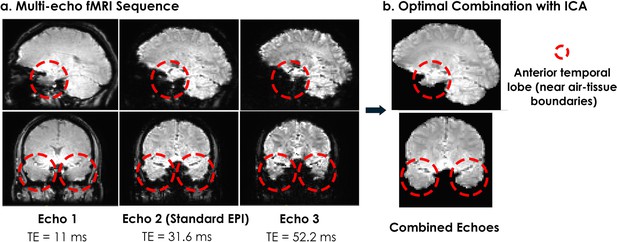
Signal quality comparison from a representative participant.
(a) The multi-echo sequence we used acquired three measurements after every radiofrequency pulse, compared to the standard single-echo EPI which acquires a single measurement (usually at a TE around 30ms). A multi-echo sequence with three echoes acquires three times as much data as the current standard single-echo approach, and accounts for differences in measured T2* across brain regions. For example, better signal is obtained at high TE values for the anterior temporal lobes, which would otherwise reveal substantial signal dropout due to susceptibility artifacts at TE = 30ms. (b) We optimally averaged the three echoes, using a method that weighs the combination of echoes based on the estimated at each voxel for each echo, and then applied ICA decomposition to remove non-BOLD noise. We found that the multi-echo approach better recovered signal from the anterior temporal lobe structures compared to the standard single-echo approach (shown in the Echo 2 column).

Pattern similarity analyses for unimodal feature runs.
(a) Contrast matrix comparing the effect of congruency on feature representations. The voxel-wise matrix averaged across unimodal runs were autocorrelated using the z-transformed Pearson’s correlation, creating a unimodal feature-level contrast matrix. We examined the average pattern similarity between unimodal features associated with congruent objects (green) compared to the same unimodal features associated with incongruent objects (yellow). (b) Pattern similarity analysis revealed an interaction between learning day and congruency in the temporal pole (TP). At baseline before crossmodal learning, there was no difference in neural similarity between unimodal features that paired to create congruent objects compared to the same unimodal features that paired to create incongruent objects. After crossmodal learning, however, there was less neural similarity between the unimodal features of pairs comprising congruent objects compared to the unimodal features of pairs comprising incongruent objects. Because congruent and incongruent objects were built from the same shapes and sounds, this result provides evidence that learning about crossmodal object associations influenced the representations of the component features in the temporal pole. There was no difference between the congruent and incongruent pairings in any other ROI (Figure 4—figure supplement 1). ** p<0.01. Error bars reflect the 95% confidence interval (n = 17).
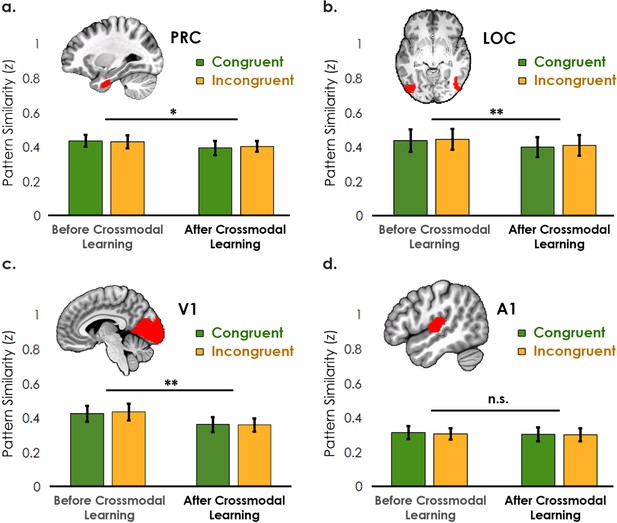
Pattern similarity analyses between unimodal features associated with congruent objects and incongruent objects, before and after crossmodal learning (analysis visualized in Figure 4 in the main text).
(a–c) Interestingly, the perirhinal cortex, LOC, and V1 – primarily visually biased regions (see main text) – reduced in pattern similarity after crossmodal learning. (d) By contrast, there was no change across learning days in A1. No region displayed a difference between congruent and incongruent feature pairings other than the temporal pole (see Figure 4). * denotes p<0.05, ** denotes p<0.01, *** denotes p<0.001. Horizontal lines denote the main effect of learning day. Error bars reflect the 95% confidence interval (n = 17).
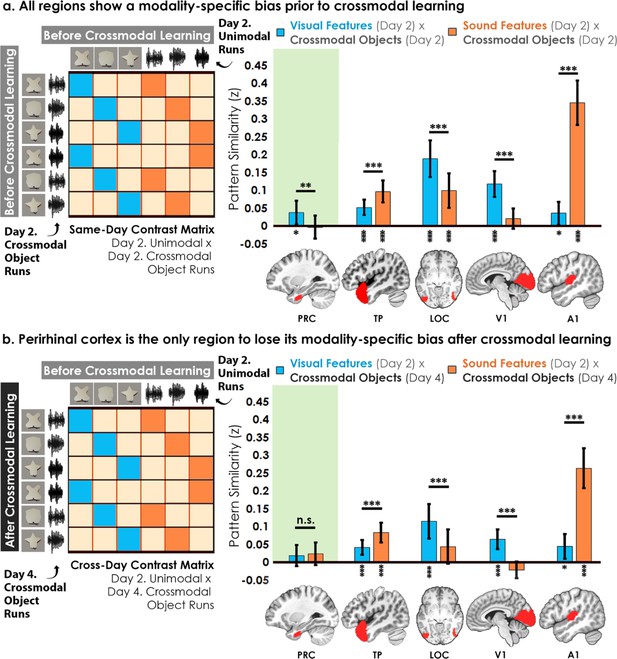
Contrast matrices and pattern similarity analyses investigating the effect of crossmodal learning on modality-specific biases.
The voxel-wise matrix for unimodal feature runs on Day 2 were correlated to the voxel-wise matrix for crossmodal object runs on (a) Day 2 and (b) Day 4, creating a contrast matrix between visual and auditory unimodal features to crossmodal objects that contained those features. We compared the average pattern similarity (z-transformed Pearson correlation) between shape (blue) and sound (orange) features across learning days. (a) Robust modality-specific feature biases were observed in all examined regions before crossmodal learning. That is, pattern similarity for each brain region was higher for one of the two modalities, indicative of a modality-specific bias. For example, pattern similarity in perirhinal cortex (PRC) preferentially tracked the visual features of the crossmodal objects, evidence of a default visual shape bias before crossmodal learning. (b) Critically, we found that perirhinal representations were transformed with experience, such that the initial visual bias was attenuated after crossmodal learning (i.e. denoted by a significant interaction, shown by shaded green regions), evidence that representations were no longer predominantly grounded in the visual modality. * p<0.05, ** p<0.01, *** p<0.001. Horizontal lines within brain regions indicate a significant main effect of modality. Vertical asterisks denote pattern similarity comparisons relative to 0. Error bars reflect the 95% confidence interval (n = 17).
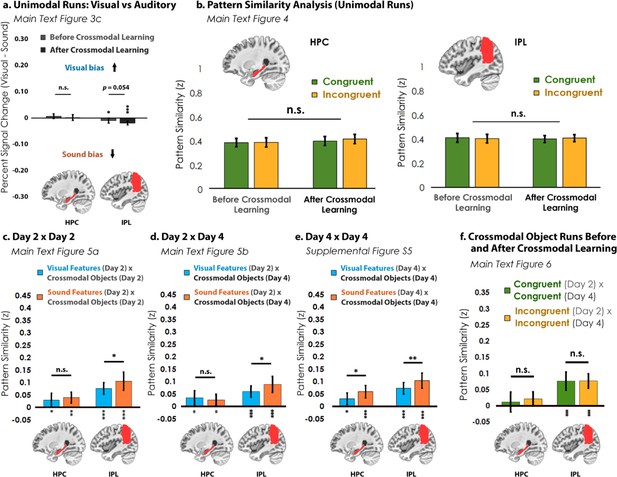
Analyses for the hippocampus (HPC) and inferior parietal lobe (IPL).
(a) In the visual vs. auditory univariate analysis, there was no visual or sound bias in HPC, but there was a bias toward sounds that increased numerically after crossmodal learning in the IPL. (b) Pattern similarity analyses between unimodal features associated with congruent objects and incongruent objects. Similar to Figure 4—figure supplement 1, there was no main effect of congruency in either region. (c) When we looked at the pattern similarity between Unimodal Feature runs on Day 2 to Crossmodal Object runs on Day 2, we found that there was significant pattern similarity when there was a match between the unimodal feature and the crossmodal object (e.g. pattern similarity >0). This pattern of results held when (d) correlating the Unimodal Feature runs on Day 2 to Crossmodal Object runs on Day 4, and (e) correlating the Unimodal Feature runs on Day 4 to Crossmodal Object runs on Day 4. Finally, (f) there was no significant pattern similarity between Crossmodal Object runs before learning correlated to Crossmodal Object after learning in HPC, but there was significant pattern similarity in IPL (p<0.001). Taken together, these results suggest that both HPC and IPL are sensitive to visual and sound content, as the (c, d, e) unimodal feature-level representations were correlated to the crossmodal object representations irrespective of learning day. However, there was no difference between congruent and incongruent pairings in any analysis, suggesting that HPC and IPL did not represent crossmodal objects differently from the component unimodal features. For these reasons, HPC and IPL may represent the convergence of unimodal feature representations (i.e. because HPC and IPL were sensitive to both visual and sound features), but our results do not seem to support these regions in forming crossmodal integrative coding distinct from the unimodal features (i.e. because representations in HPC and IPL did not differentiate the congruent and incongruent conditions and did not change with experience). * p<0.05, ** p<0.01, *** p<0.001. Asterisks above or below bars indicate a significant difference from zero. Horizontal lines within brain regions in (a) reflect an interaction between modality and learning day, whereas horizontal lines within brain regions in reflect main effects of (b) learning day, (c–e) modality, or (f) congruency. Error bars reflect the 95% confidence interval (n = 17).

The voxel-wise matrix for Unimodal Feature runs on Day 4 were correlated to the voxel-wise matrix for Crossmodal Object runs on Day 4 (see Figure 5 in the main text for an example).
We compared the average pattern similarity (z-transformed Pearson correlation) between shape (blue) and sound (orange) features specifically after crossmodal learning. Consistent with Figure 5b, perirhinal cortex was the only region without a modality-specific bias. Furthermore, perirhinal cortex was the only region where the representations of both the visual and sound features were not significantly correlated to the crossmodal objects. By contrast, every other region maintained a modality-specific bias for either the visual or sound features. These results suggest that perirhinal cortex representations were transformed with experience, such that the initial visual shape representations (Figure 5a) were no longer grounded in a single modality after crossmodal learning. Furthermore, these results suggest that crossmodal learning formed an integrative code different from the unimodal features in perirhinal cortex, as the visual and sound features were not significantly correlated with the crossmodal objects. * p<0.05, ** p<0.01, *** p<0.001. Horizontal lines within brain regions indicate a significant main effect of modality. Vertical asterisks denote pattern similarity comparisons relative to 0. Error bars reflect the 95% confidence interval (n = 17).
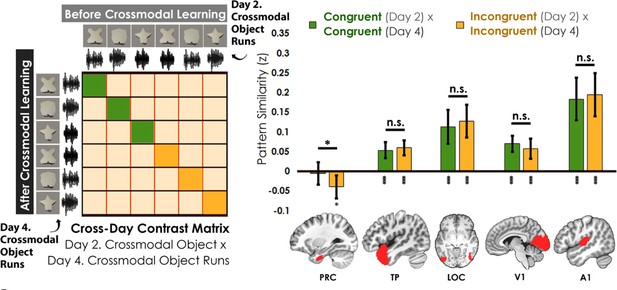
Contrast matrix shown on the left panel, with actual results shown on the right panel.
We compared the average pattern similarity across learning days between crossmodal object runs on Day 2 with crossmodal object runs on Day 4 (z-transformed Pearson correlation). We observed lower average pattern similarity for incongruent objects (yellow) compared to congruent (green) objects in perirhinal cortex (PRC). These results suggest that perirhinal cortex differentiated congruent and incongruent objects constructed from the same features. Furthermore, pattern similarity was never above 0 for the perirhinal cortex. By contrast, there was no significant difference between congruent and incongruent objects in any other examined region, and pattern similarity was always above 0. * denotes p<0.05, ** denotes p<0.01, *** denotes p<0.001. Horizontal lines within brain regions denote a main effect of congruency. Vertical asterisks denote pattern similarity comparisons relative to 0. Error bars reflect the 95% confidence interval (n = 17).